The Preregistration Prescriptiveness Trade-Off and Unknown Unknowns in Science
Comments on Van Drimmelen (2023)
Abstract
I discuss Van Drimmelen’s (2023) Metascience2023 presentation on researchers’ decision making during the research process. In particular, I consider his evidence that researchers’ discretion over research decisions is unavoidable when they follow research plans that are either overdetermined (i.e., too prescriptive) or underdetermined (i.e., too vague). I argue that this evidence points to a prescriptiveness trade-off when writing preregistered plans: All other things being equal, plans that are more prescriptive are more likely to result in deviations that turn their confirmatory tests into exploratory tests, and plans that are less prescriptive are more likely to result in confirmatory tests that are susceptible to questionable research practices. I also consider Van Drimmelen’s idea that researchers may make unconscious, implicit decisions during the research process. I relate these implicit decisions to Rumsfeld’s (2002) concept of unknown unknowns: “the things we don’t know we don’t know”! I argue that scientists can report their known knowns (what they know they did and found), and they can be transparent and speculative about their known unknowns (what they know they didn’t do and may find), but that they can’t say much about their unknown unknowns (including their unconscious, implicit decisions) because, by definition, they don’t know what they are! Nonetheless, I think that it’s important to acknowledge unknown unknowns in science because doing so helps to contextualise research efforts as being highly tentative and fallible.
Introduction
The videos of the presentations at the Metascience2023 conference were recently made available here. One that caught my eye is by Tom van Drimmelen, titled “Researchers’ decision making: Navigating ambiguity in research practice”:
Here, I summarise Van Drimmelen’s excellent work and share my thoughts on his ideas.
What is Researcher Discretion?
Van Drimmelen (2023) explained that, during the research process, researchers have discretion over numerous decisions that they make about their methodology and analyses. During the data analysis stage, this researcher discretion may be perceived negatively as researcher degrees of freedom that is susceptible to researcher bias and questionable research practices (John et al., 2012; Simmons et al., 2011).
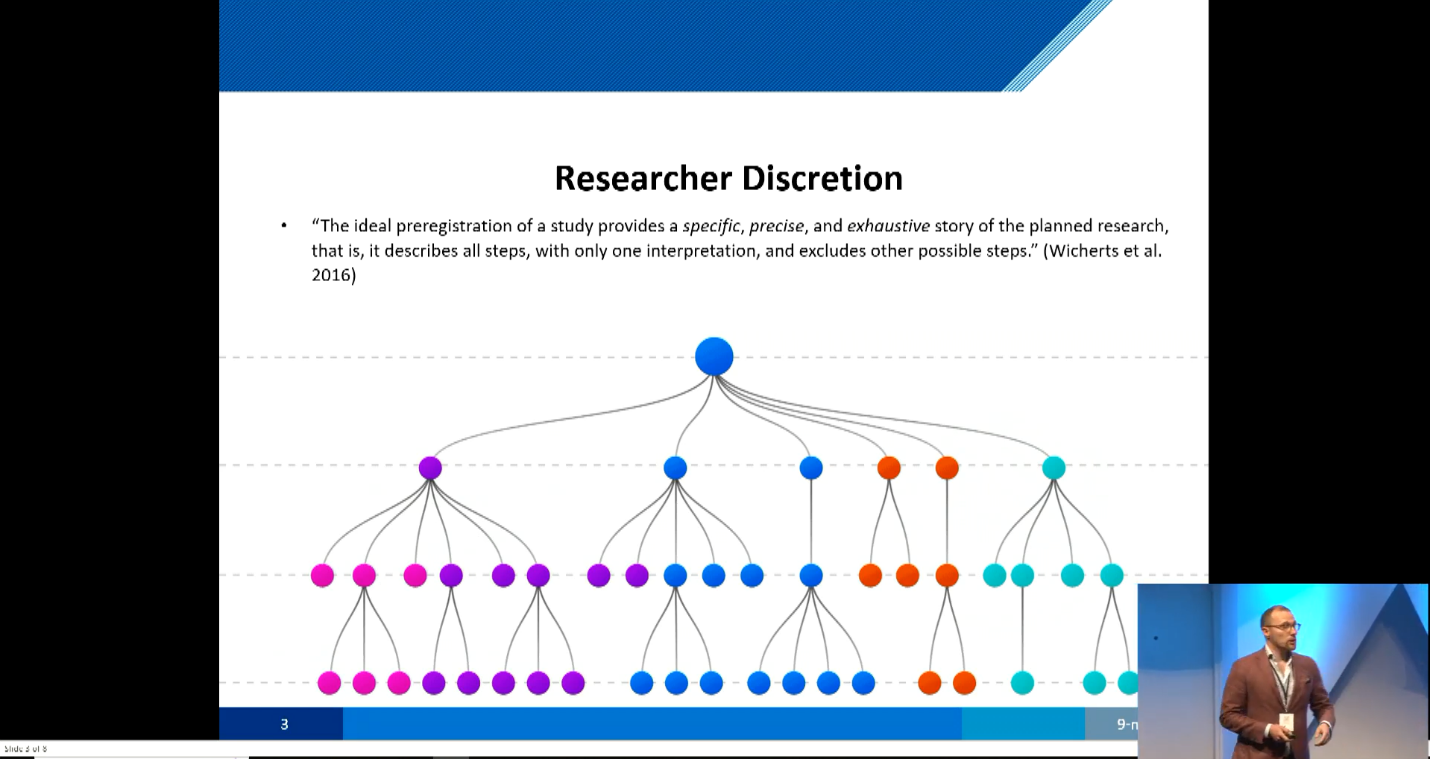
Researcher Discretion Emerges in Both Over- and Under-Determined Research Plans
In theory, it is possible to reduce and/or reveal researcher discretion during data analyses by preregistering one or more methodological and analytical research paths. However, Van Drimmelen (2023) and colleagues were interested in how researcher discretion operates in practice. To investigate, they conducted an ethnographic study in which they observed researcher discretion in two different research groups. They found that researcher discretion emerged when researchers used two categories of research plan.
1. Underdetermined plans: Researcher discretion emerged when research plans were not precise enough to determine specific actions for the researchers. In this case, researchers needed to use their own discretion to fill in the gaps of the research plan and figure out how to proceed. Researchers often find themselves in this situation because preregistered research plans are often vague (e.g., Bakker et al., 2020; Heirene et al., 2021; Van den Akker et al., 2023).
2. Overdetermined plans: Researcher discretion also emerged when there were unforeseen changes in the research situation that made the original research plan undesirable or impossible, necessitating an alternative research approach. Again, this situation is common, because most researchers do not possess “godlike planning abilities” (Navarro, 2020). To attempt to address this issue, researchers can preregister “if…then” contingencies (i.e., decision trees) that accommodate anticipated changes in the research situation (Nosek et al., 2018, p. 2602). In addition, it may be possible to update a preregistered analysis plan prior to the data analysis in order to take account of unanticipated events (Nosek et al., 2019, p. 817). However, it is often the case that unanticipated events arise during data analyses. As Reinhart (2015, p. 95) explained (paraphrasing Helmuth von Moltke), “no analysis plan survives contact with the data.” In these cases, researchers either need to stick to an inadequate preregistered analysis plan or deviate and adapt their approach to address the current situation. The evidence shows that researchers often deviate from their preregistered plans (e.g., Abrams et al., 2020; Claesen et al., 2021; Heirene et al., 2021).
A Prescriptiveness Trade-Off in Preregistered Plans
Van Drimmelen’s (2023) work shows us that researchers are forced to either deviate from overdetermined plans to overcome unforeseen events or fill in the gaps of vague, underdetermined plans. Hence, researcher discretion emerges in the case of both over- and under-determined plans. As Van Drimmelen (2023) put it, “researcher discretion is an integral and unavoidable part of the research practice.”
I think Van Drimmelen’s (2023) evidence also points to a trade-off between the prescriptiveness of preregistered research plans and the feasibility of their implementation in an unpredictable world. As preregistered plans become more precise and prescriptive, there is a greater chance that researchers will need to deviate from them in order to accommodate unforeseen and/or uncontrolled events that occur during the implementation of the plan. For example, researchers are more likely to deviate from a plan to test “300 participants” than they are to deviate from a less prescriptive plan to test “around 300 participants,” because uncontrolled events (e.g., the number of participant exclusions during data analysis) may result in a final sample size that is only close to 300 participants and not exactly 300 participants (e.g., Claesen et al., 2021, pp. 6-7). Consequently, as preregistered plans become more prescriptive, they are more likely to result in deviations that turn their planned confirmatory tests into unplanned exploratory tests.1 Of course, preregistration continues to distinguish between confirmatory and exploratory tests in this situation, and it prevents exploratory tests from being falsely portrayed as confirmatory tests. Nonetheless, it remains the case that, as plans for confirmatory tests become more prescriptive, the actual implementation of these confirmatory tests in the real world becomes less likely.
On the other hand, as preregistered plans become vaguer and less prescriptive, confirmatory hypothesis tests will become not only more feasible to achieve without deviation, but also more susceptible to researcher degrees of freedom and questionable research practices. For example, it is more feasible to test “around 300 participants” than to test exactly “300 participants.” However, the first sampling plan does not prevent the questionable research practice of optional stopping (John et al., 2021; Simmons et al., 2011), whereas the second, more prescriptive plan does.
In summary, researchers need to consider a prescriptiveness trade-off when writing their preregistered plans: All other things being equal, plans that are more prescriptive are more likely to result in deviations that turn their confirmatory tests into exploratory tests, and plans that are less prescriptive are more likely to result in confirmatory tests that are susceptible to questionable research practices. Hence, researchers face a difficult choice between prescriptive confirmatory tests that they are less likely to carry out in practice and vague confirmatory tests that are more prone to questionable research practices.
Researchers Sometimes Make Implicit Decisions
Van Drimmelen and colleagues (2023) also identified another interesting issue in their work. At times, they found it difficult to identify researcher discretion because there were multiple potential methodological or analytical approaches available that researchers did not consider. In other words, sometimes researchers were not aware that they were making decisions!
This idea of unconscious and implicit research decisions reminds me of something that Donald Rumsfeld, the United States Secretary of Defence, said at a press briefing in 2002:
There are known knowns; there are things we know we know. We also know there are known unknowns; that is to say we know there are some things we do not know. But there are also unknown unknowns—the ones we don’t know we don’t know. (Wikipedia, 2023)
In the context of Figure 1, our known knowns are the path or paths that we reported following in our research (e.g., the green paths). In these cases, we know what we did, and we know what we found as a result. In contrast, our known unknowns are the paths that we actively decided not to follow (e.g., all the other paths in Figure 1). We know these paths exist, but we don’t yet know what results lie at the end of them.
But what about our unknown unknowns? These paths don’t appear in Figure 1! They relate to decisions that we didn’t even consider, at least not at a conscious level. For example, we may not have considered the time of day of testing, the ambient temperature, or a host of other unspecified variables that may be influential on our results. Instead, we unconsciously relegated these unknown unknowns to a ceteris paribus clause (i.e., “all other things being equal”), which assumes that no other influential factors are at play (Meehl, 1990). The problem is that our unconscious and implicit decision to assume the irrelevance of these factors may be wrong! Indeed, the history of science is full of cases in which factors that were not initially considered to be important subsequently turned out to be crucial moderators or boundary conditions of effects (for some examples, see Firestein, 2016).
We Can’t Be Transparent About Unknown Unknowns, But We Should Acknowledge They Exist!
Van Drimmelen (2023) concluded that “we might not be able to be effectively transparent because we don’t know which decisions we’ve actually made” (my emphasis). In other words, our lack of awareness about our potentially important implicit decisions means that we can’t be transparent about them: We can report our known knowns, and we can be transparent and speculative about our known unknowns, but we can’t say much about our unknown unknowns because we don’t know what they are! By definition, as soon as we start to speculate on a potentially influential factor in our research, it becomes a known unknown about which we need to make conscious decisions!
Despite our inability to specify our unknown unknowns, Van Drimmelen’s (2023) work reminds us that it’s important to acknowledge this type of ignorance in science because it helps to contextualise our research efforts as being highly tentative and fallible steps in a collective process of discovery and theory development. Acknowledging our unknown unknowns also helps to address recent calls for greater intellectual humility and modesty in science (Hoekstra & Vazire, 2021; Ramsey, 2021).
I’ll end with a nice example of scientists acknowledging unknown unknowns. Here’s Errington et al. (2021, p. 12) discussing the challenges of assessing replicability in preclinical cancer biology:
It might also be that, in some cases, a failure to replicate was caused by the replication team deviating from the protocol in some way that was not recognized, or that a key part of the procedure was left out of the protocol inadvertently. It is also possible that the effect reported in the original paper depended on methodological factors that were not identified by original authors, the replication team, or any other experts involved in the peer review of the original paper or the Registered Report.
References
Abrams, E., Libgober, J., & List, J. A. (2020). Research registries: Facts, myths, and possible improvements (No. w27250). National Bureau of Economic Research. https://doi.org/10.3386/w27250
Bakker, M., Veldkamp, C. L., van Assen, M. A., Crompvoets, E. A., Ong, H. H., Nosek, B. A.,…& Wicherts, J. M. (2020). Ensuring the quality and specificity of preregistrations. PLoS Biology, 18(12), e3000937. https://doi.org/10.1371/journal.pbio.3000937
Claesen, A., Gomes, S., Tuerlinckx, F., Vanpaemel, W., & Leuven, K. U. (2021). Comparing dream to reality: An assessment of adherence of the first generation of preregistered studies. Royal Society Open Science, 8(10), 1–11. https://doi.org/10.1098/rsos.211037
Errington, T. M., Denis, A., Perfito, N., Iorns, E., & Nosek, B. A. (2021). Reproducibility in cancer biology: Challenges for assessing replicability in preclinical cancer biology. Elife, 10, Article e67995. https://doi.org/10.7554/eLife.67995
Fife, D., & Rodgers, J. L. (2019). Moving beyond the "replication crisis": Understanding the exploratory/confirmatory data analysis continuum. PsyArXiv. https://doi.org/10.31234/osf.io/5vfq6
Firestein, S. (2016, February 14). Why failure to replicate findings can actually be good for science. LA Times. https://www.latimes.com/opinion/op-ed/la-oe-0214-firestein-science-replication-failure-20160214-story.html
Heirene, R., LaPlante, D., Louderback, E. R., Keen, B., Bakker, M., Serafimovska, A., & Gainsbury, S. M. (2021). Preregistration specificity & adherence: A review of preregistered gambling studies & cross-disciplinary comparison. PsyArXiv. https://psyarxiv.com/nj4es/
Hoekstra, R., & Vazire, S. (2021). Aspiring to greater intellectual humility in science. Nature Human Behaviour, 5(12), 1602-1607. https://doi.org/10.1038/s41562-021-01203-8
John, L. K., Loewenstein, G., & Prelec, D. (2012). Measuring the prevalence of questionable research practices with incentives for truth telling. Psychological Science, 23(5), 524–532. https://doi.org/10.1177/0956797611430953
Meehl, P. E. (1990). Appraising and amending theories: The strategy of Lakatosian defense and two principles that warrant it. Psychological Inquiry, 1(2), 108-141. https://doi.org/10.1207/s15327965pli0102_1
Navarro, D. (2020, September 23). Paths in strange spaces: A comment on preregistration. PsycArXiv. https://doi.org/10.31234/osf.io/wxn58
Nosek, B. A., Beck, E. D., Campbell, L., Flake, J. K., Hardwicke, T. E., Mellor, D. T., van ‘t Veer, A. E., & Vazire, S. (2019). Preregistration is hard, and worthwhile. Trends in Cognitive Sciences, 23, 815–818. https://doi.org/10.1016/j.tics.2019.07.009
Ramsey, R. (2021). A call for greater modesty in psychology and cognitive neuroscience. Collabra: Psychology, 7(1), 24091. https://doi.org/10.1525/collabra.24091
Reinhart, A. (2015). Statistics done wrong: The woefully complete guide. No Starch Press.
Simmons, J. P., Nelson, L. D., & Simonsohn, U. (2011). False-positive psychology: Undisclosed flexibility in data collection and analysis allows presenting anything as significant. Psychological Science, 22(11), 1359–1366. https://doi.org/10.1177 /0956797611417632
Van den Akker, O., Bakker, M., van Assen, M. A. L. M., Pennington, C. R., Verweij, L., Elsherif, M. M.,…Wicherts, J. M. (2023, May 10). The effectiveness of preregistration in psychology: Assessing preregistration strictness and preregistration-study consistency. PsyArXiv. https://doi.org/10.31222/osf.io/h8xjw
Van Drimmelen, T., Slagboom, N., Reis, R., Bouter, L., Van der Steen, J. (2023, May 9). Researchers’ decision making: Navigating ambiguity in research practice. Metascience2023, Washington, DC. Abstract: https://metascience.info/events/researchers-decision-making-navigating-ambiguity-in-research-practice/ Preregistration: https://osf.io/tqwgp/
Wikipedia. (2023, March 16). There are unknown unknowns. Wikipedia. https://en.wikipedia.org/wiki/There_are_unknown_unknowns
Endnotes
1. Here, I define confirmatory tests as planned tests, and exploratory tests as unplanned tests. This conceptualization is consistent with the frequentist rationale for preregistration, according to which unplanned tests undermine assumptions regarding Type I error rates (Nosek et al., 2019, p. 816). I acknowledge that there are other definitions for “confirmatory” and “exploratory”, including definitions that view them as existing at opposite ends of a continuum rather than as discrete categories (e.g., Fife & Rodgers, 2019). According to this continuum perspective, larger deviations from preregistered plans result in “more exploratory” tests than smaller deviations. I don’t subscribe to this continuum perspective, because it is unclear how to formally assess some deviations as being “larger” or “smaller” than others. I agree that research studies can be described as being more confirmatory or exploratory, because they can contain a greater proportion of planned or unplanned tests. However, in my view, the tests themselves can only be either confirmatory or exploratory, because they can only be either planned or unplanned.
Acknowledgements
I’m grateful to Tom van Drimmelen for his comments on an earlier version of this article.
PDF Download
You can download a PDF version of this article here.
Reference
The reference for this article is:
Rubin, M. (2023, June 7). The preregistration prescriptiveness trade-off and unknown unknowns in science: Comments on Van Drimmelen (2023). Critical Metascience: MetaArXiv. https://doi.org/10.31222/osf.io/3t7pc
Hello Mark, I liked your post and agree with most of it. My only disagreement is with the notion that preregistered confirmatory tests become exploratory whenever researchers deviate from their preregistration. To me, there is a difference between a planned but deviated test and an unplanned test. I agree with you that confirmation and exploration are distinguished based on whether the test was planned, but I think that a deviated plan is still a plan, and so deviated preregistrations are still confirmatory.
However, not all confirmatory tests are equal. Firstly, a stricter preregistration prohibits more possible scenarios and thus can confirm more than a vaguer preregistration. Secondly, a confirmatory test’s confirmation decreases as the amount of deviations increase. Thus, I would rephrase the preregistration trade-off you have identified as one involving confirmation: assuming that the goal of researchers is to confirm their theories as much as they can, they are faced with a trade-off between 1) strict preregistrations that has more potential confirmation which can decrease if deviations are made, and 2) vague preregistrations that has less potential confirmation which can also decrease if deviations are made but such deviations are less likely. Basically, researchers can choose to make big bets (strict preregistrations) with potentially big pay-offs (strong confirmation) or small bets (vague preregistrations) with potentially small pay-offs (weak confirmation).
Hi Mark, great post! A lot of this resonates with my own experiences with preregistration.
I'd tend to think that the two scenarios of a deviation from a strict preregistration or an unanticipated decision after a vague preregistration tend to have pretty similar consequences for the credibility of the analysis subsequently presented. I.e., in both cases there is the possibility (albeit not the certainty) that knowledge about the results produced by different analysis choices affected which analysis the researcher decided to report.
Personally, I edge towards the strict preregistration route, but for pretty banal reasons. My experience has been that when a preregistration leaves a particular decision unstated, it's pretty easy for that to fall between the cracks in a communication sense. The researcher might not "click" that they actually had to make a decision, or they might forget to write down that this happened, and the reviewers may not realise that a decision needed making. In contrast, when a preregistration said X but the study did Y, it's a little more obvious to all concerned.
By the way, the idea of trade-offs makes me think of the one applying to data analysis plans created before versus after we've seen the data. When we create the analysis plan first (as in a preregistration), this has the advantage of ruling out the possibility of the observed values of the statistics from affecting which statistics we choose to report, this being a potential source of bias. However, this plan can't use other information we obtain after collecting data (e.g., knowledge about distributional assumption violations). In contrast, a plan created or modified after collecting data can take into account useful information from the data itself, but comes with the possibility that analysis decisions are consciously or unconsciously affected by knowledge of what substantive results they produce.
I don't think one could make a principled argument that either of these options is *in general* better than the other (unless one were a rabid predictivist or something). It depends on the situation. But when peer reviewing a preregistered study I do quite like being able to read about *both*!