The inflation of Type I error rates is thought to be one of the causes of the replication crisis. Questionable research practices such as p-hacking are thought to inflate Type I error rates above their nominal level, leading to unexpectedly high levels of false positives in the literature and, consequently, unexpectedly low replication rates. In this article, I offer an alternative view. I argue that questionable and other research practices do not usually inflate relevant Type I error rates. I illustrate my argument with respect to model misspecification, multiple testing, selective inference, forking paths, exploratory analyses, p-hacking, optional stopping, double dipping, and HARKing.
Type I Error Rates Cover Statistical Errors, Not Theoretical Errors
I begin with an introduction to Type I error rates that distinguishes them from theoretical errors. Statistical errors only refer to random sampling error. In contrast, theoretical errors refer to a wide range of misinterpretations about (a) theory (e.g., misinterpreted theoretical rationales, hypotheses, and predictions), (b) methodology (e.g., misspecified participant populations, sampling procedures, testing conditions, stimuli, manipulations, measures, controls, etc.), (c) data (e.g., misspecified procedures for data selection, entry, coding, cleaning, aggregation, etc.), and (d) analyses (e.g., misspecified statistical models and assumptions, misinterpreted statistical results). Consistent with several others, I note that theoretical errors are not covered by the Type I error rate, and they can have a larger impact than Type I errors (Bolles, 1962; Chow, 1998; Cox, 1958; Hager, 2013; Meehl, 1978, 1997; Neyman, 1950).
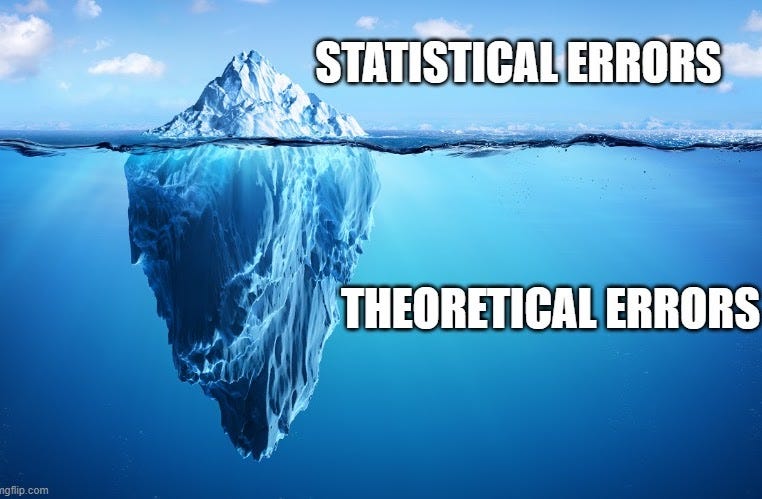
Type I Error Rate Inflation is Uncommon and Easily Identified and Resolved
I then consider how Type I error rates become inflated. During significance testing, each statistical inference is assigned a nominal Type I error rate. Type I error rate inflation occurs if the actual Type I error rate for that inference is higher than its nominal error rate. The actual Type I error rate is calculated using the formula 1 - (1 - α)k, in which k is the number of significance tests that are used to make the statistical inference. I argue that the actual Type I error rate is not usually inflated above the nominal rate because researchers usually adjust their significance threshold to maintain it at its nominal level and, when they don't, the error rate inflation is transparent and easily resolved because k is known by readers, and they can make their own adjustments if necessary.
I argue that k must be known by readers because researchers must formally associate each of their statistical inferences with one or more significance tests, and k is the number of those tests. I stress that k is not the number of tests that researchers conduct in their studies, including those that they conduct and then fail to report. Instead, k is the number of tests that are used to make a particular statistical inference about a specified null hypothesis.
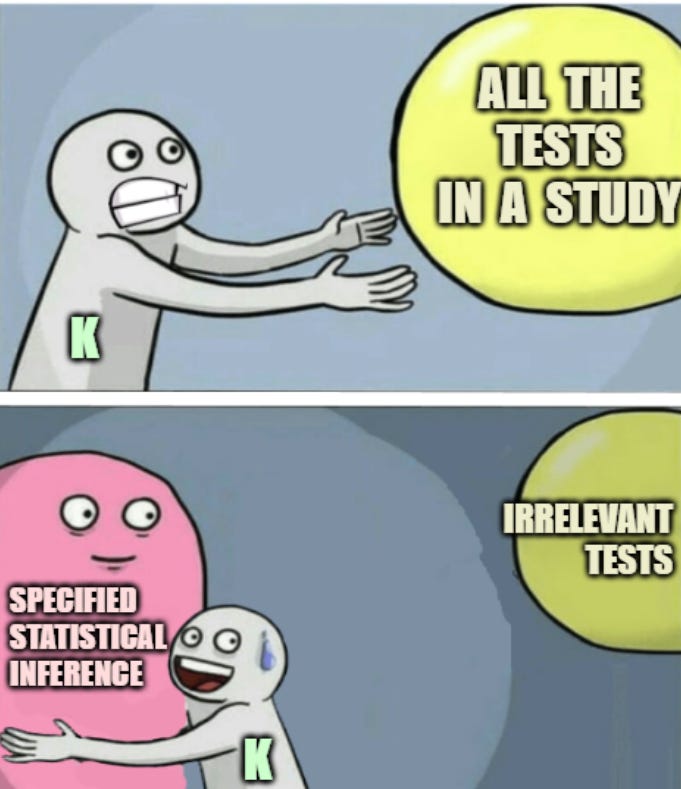
What About the Evidence for Type I Error Rate Inflation?
I also consider the evidence for Type I error inflation and conclude that two problems threaten its validity.
First, evidence of Type I error inflation tends to confound statistical inferences about individual null hypotheses (e.g., H1) with statistical inferences about joint null hypotheses (e.g., "H1 & H2 & H3...& H20"). For example, simulations of actual Type I error rates may compute the familywise error rate for a joint null hypothesis and then apply that error rate to individual null hypotheses, claiming that, because the familywise error rate is, for example, .642, there is a .642 chance of incorrectly rejecting each individual null hypothesis. This reasoning is widely acknowledged to be incorrect (Armstrong, 2014, p. 505; Cook & Farewell, 1996, pp. 96–97; Fisher, 1971, p. 206; García-Pérez, 2023, p. 15; Greenland, 2021, p. 5; Hewes, 2003, p. 450; Hurlbert & Lombardi, 2012, p. 30; Matsunaga, 2007, p. 255; Molloy et al., 2022, p. 2; Parker & Weir, 2020, p. 564; Parker & Weir, 2022, p. 2; Rothman, 1990, p. 45; Rubin, 2017, pp. 271–272; Rubin, 2020, p. 380; Rubin, 2021a, 2021b, pp. 10978-10983; Savitz & Olshan, 1995, p. 906; Senn, 2007, pp. 150-151; Sinclair et al., 2013, p. 19; Tukey, 1953, p. 82; Turkheimer et al., 2004, p. 727; Veazie, 2006, p. 809; Wilson, 1962, p. 299; for the relevant quotations, please see Appendix B here). If a researcher makes a statistical inference based on a single test of a single individual hypothesis using a p < .050 significance threshold, then their actual Type I error rate for that inference will be .050 regardless of whether they make 20 or a million other statistical inferences and even if their statistical result for that inference is the only significant result that they obtain or report.
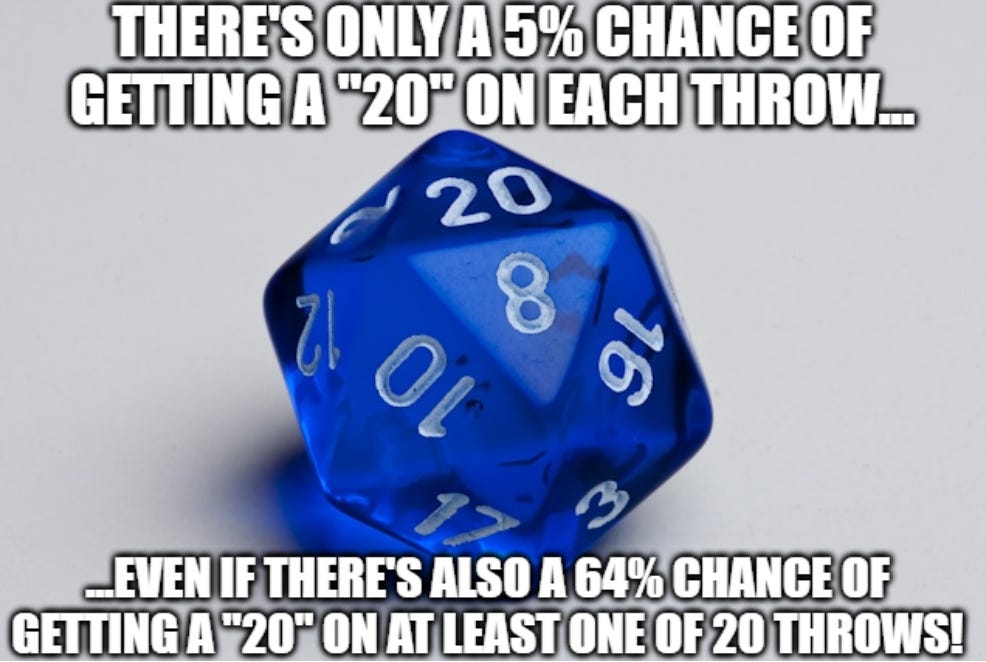
Second, evidence of Type I error rate inflation may depend on a fallacious comparison between (a) the probability of rejecting a null hypothesis when it is true and (b) the probability of a null hypothesis being true when it is rejected (Pollard & Richardson, 1987). The first probability is equivalent to a frequentist Type I error rate: Pr(reject H0 ; H0 is true). However, the second probability does not provide an appropriate benchmark against which to judge Type I error rate inflation because it represents a conditional posterior probability about the truth of the null hypothesis given its rejection: Pr(H0 is true|reject H0). Hence, showing that Pr(H0 is true|reject H0) > Pr(reject H0 ; H0 is true) does not provide a valid demonstration of Type I error inflation. Instead, it demonstrates the Bayesian inversion fallacy because it confuses the unconditional probability of rejecting a true null hypothesis with the conditional probability that a null hypothesis is true given that it has been rejected (Gigerenzer, 2018; Greenland et al., 2016; Mayo & Morey, 2017; Pollard & Richardson, 1987).
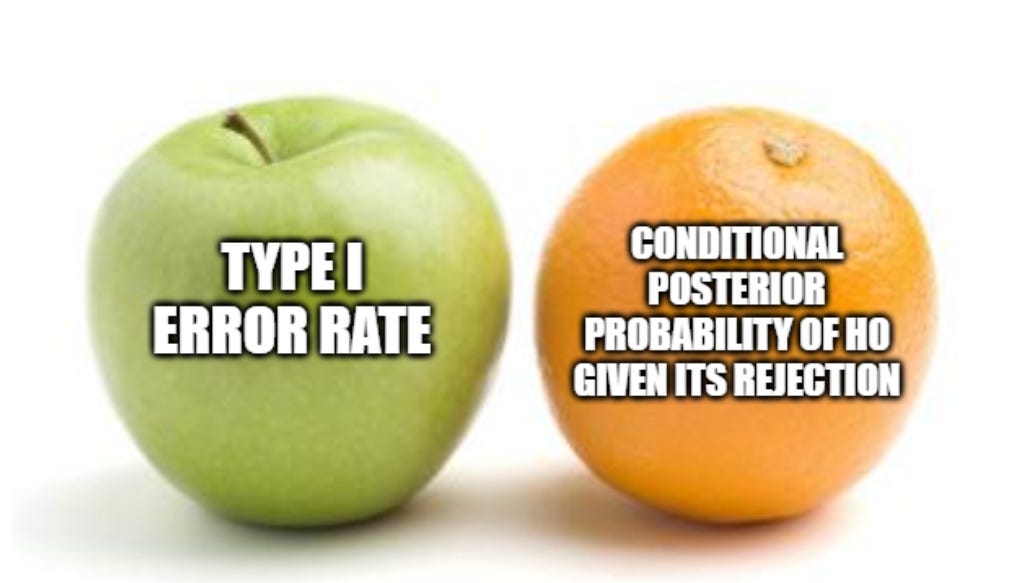
The Replication Crisis May Be Due To Unexpected Theoretical Errors Rather Than Unexpected Statistical Errors
I conclude that Type I error rate inflation may not be a major contributor to the replication crisis. Certainly, some failed replications may be due to Type I errors in original studies. However, actual Type I error rates are rarely inflated above their nominal levels, and so the level of Type I errors in a field is liable to be around that field’s conventional nominal level (see also Neyman, 1977, p. 108). Hence, Type I error rate inflation cannot explain unexpectedly low replication rates.
In contrast, theoretical errors may be higher than expected. In particular, unacknowledged misinterpretations of theory, methodology, data, and analyses may all inflate theoretical errors above their “nominal” expected level, resulting in incorrect theoretical inferences and unexpectedly low replication rates. For example, researchers may assume a higher degree of theoretical equivalence between an original study and a “direct” replication than is warranted. A failed replication may then represent the influence of an unrecognized “hidden moderator” that produces a true positive result in the original study and a true negative result in the replication study. Of course, scientists should attempt to specify and investigate such hidden moderators in future studies (Klein et al., 2018, p. 482). Nonetheless, ignoring hidden moderators does not mitigate their deleterious impact on replicability!
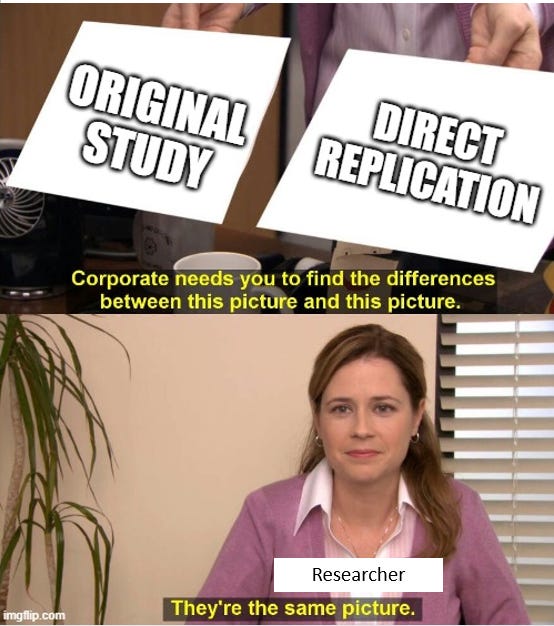
Further Information
The Article
Rubin, M. (2024). Type I error rates are not usually inflated. Journal of Trial & Error. https://doi.org/10.36850/4d35-44bd
Related Work
Rubin, M. (2022). Green jelly beans and studywise error rates: A “theory first” response to Goeman (2022). PsyArXiv. https://doi.org/10.31234/osf.io/kvynf
Rubin, M. (2021). There’s no need to lower the significance threshold when conducting single tests of multiple individual hypotheses. Academia Letters, Article 610. https://doi.org/10.20935/AL610
Rubin, M. (2021). When to adjust alpha during multiple testing: A consideration of disjunction, conjunction, and individual testing. Synthese, 199, 10969–11000. https://doi.org/10.1007/s11229-021-03276-4
Rubin, M. (2017). Do p values lose their meaning in exploratory analyses? It depends how you define the familywise error rate. Review of General Psychology, 21(3), 269-275. https://doi.org/10.1037/gpr0000123
That rare article that coherently explains one’s disorganized thinking on the topic. Congrats!